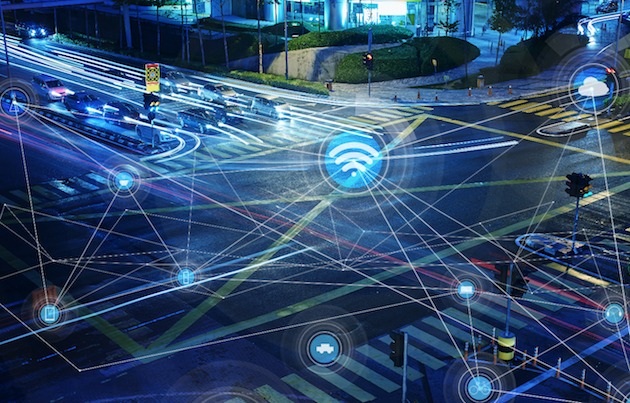
New and Improved VMware Premier Support Offering: Announced at VMworld US 2019
August 26, 2019
Taking Web Animations One Step Further With Powerful S1-Lottie
August 26, 2019Google AdSense helps countless businesses make money from their content by placing advertisements–and their recommendation system plays a huge role in this work. From the beginning, the team operated a batch-based data processing pipeline for the recommendation system, but like many Google Cloud customers we work with, they saw a lot of opportunity in migrating to a stream processing model which could enable AdSense publishers to receive real-time recommendations for their setup. As a result, in 2014, the AdSense publisher optimization team began exploring how to change their underlying data processing system.
In this post, we will walk through the technical details of how the AdSense publisher optimizationdata engineering team made the switch, and what they learned. Although the AdSense team used FlumeJava, an internal Google tool, their lessons learned are directly applicable to Google Cloud customers since FlumeJava is the same technology Google Cloud customers know as Cloud Dataflow. Today, these technologies share the majority of their code base, and further unification of FlumeJava and Cloud Dataflow is part of ongoing engineering efforts.
The original pipeline
Prior to making the change in 2014, the team’s original pipeline would extract data from several repositories, carry out any data transformations required, and then join the various data points using a common key. These new denormalized rows of data would then be used to generate AdSense’s recommendations. Once the batch run had completed, the recommendations could be communicated to the publishers. As you might expect, the pipeline needed to process a large amount of data on every run, so running the pipeline frequently was not a practical option. That meant it wasn’t suited for publishing recommendations in real time.
Moving to a streaming pipeline
The streaming pipeline that was developed went through several evolutions. In the first iteration, not every source of data was converted to be an unbounded (streaming) source, creating a pipeline that mixed bounded lookup data which was infrequently updated with the unbounded stream of data.
Blending real time and historic data sources in a combination of batch and stream is an excellent first step in migrating your environment towards real-time, and in some cases will effectively address the incremental capabilities the use case called for. It is important to make use of technologies that can blend both batch and stream processing, enabling users to move different aspects of their workloads between stream and batch until they find the right blend of speed, comfort, and price.
Initial version, unbounded data sources
In order to convert the primary sources of data from batch reads to streamed updates, the pipeline consumed the updates by connecting to a stream of change data capture (CDC) information coming from the data sources.
Initial version, with bounded lookup data sources