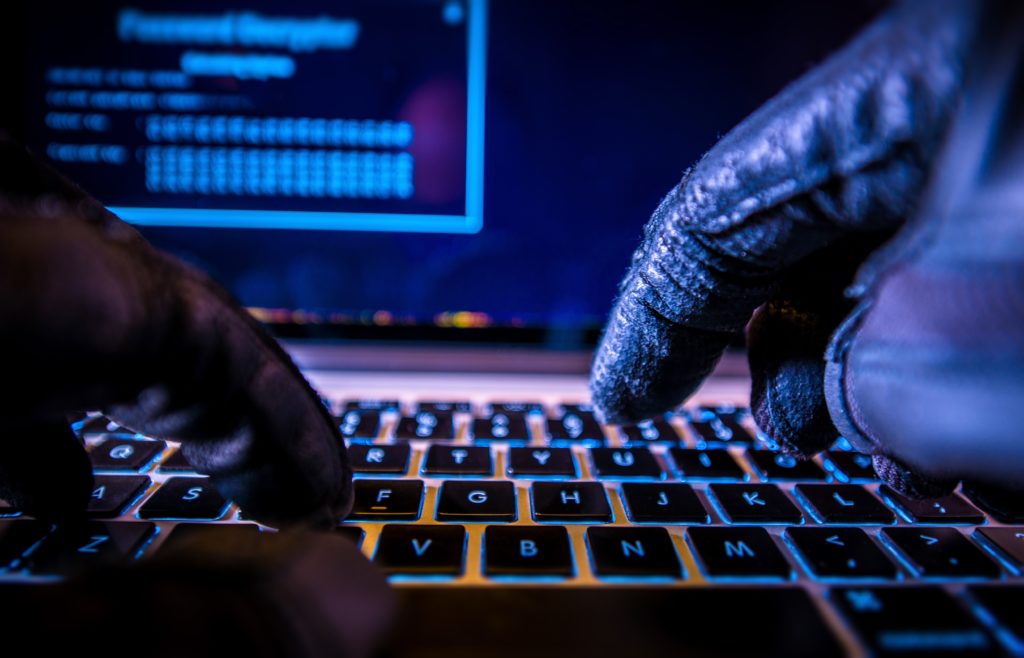
Scale your containerized application with Microsoft Azure Kubernetes Service (AKS)
August 21, 2019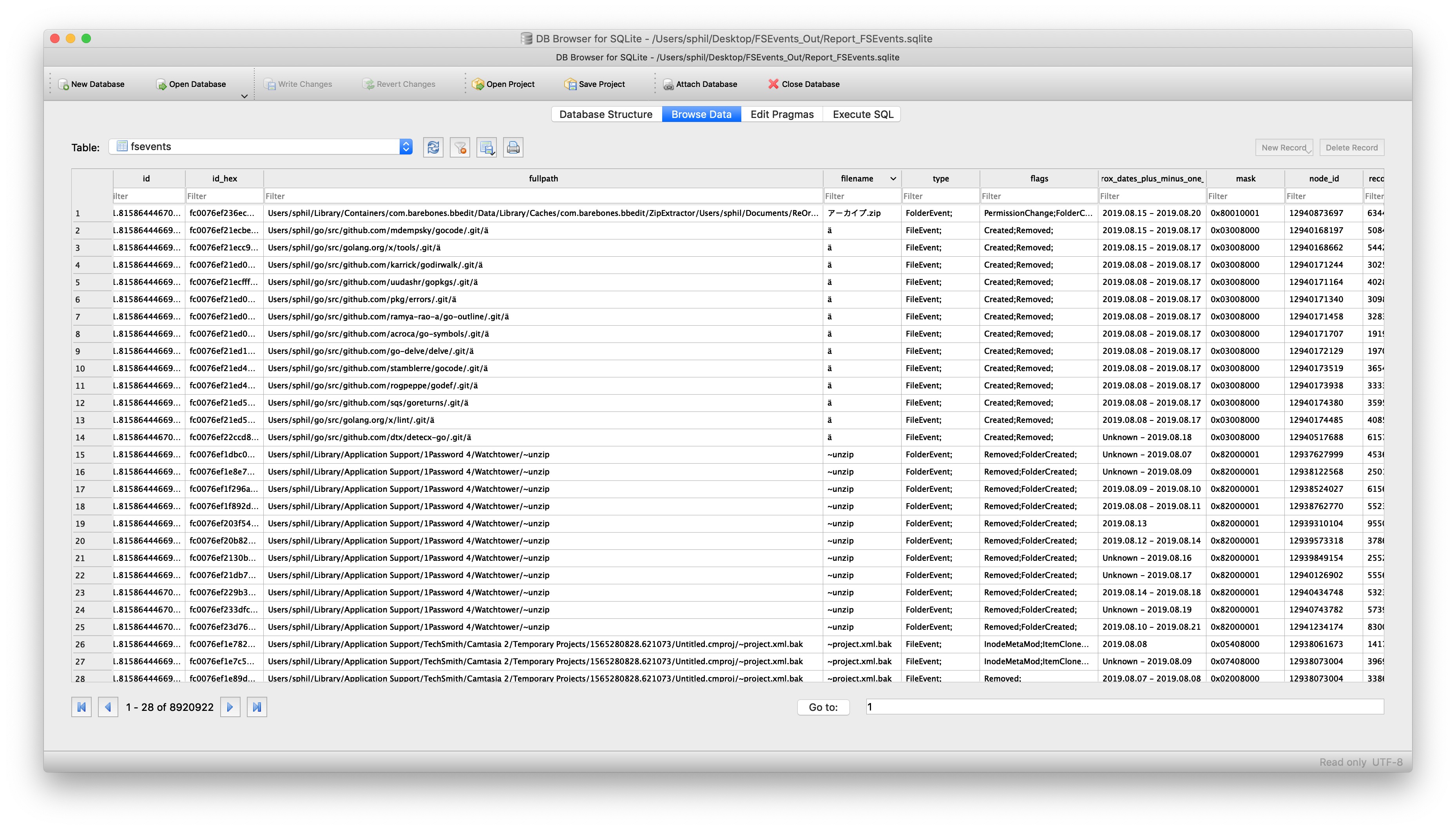
macOS Incident Response | Part 1: Collecting Device, File & System Data
August 21, 2019To cut time on managing a data warehouse, it helps to automate the system engineering work away from the analytics work, like BigQuery enables. Once those functions are separated, the analytics work can take center stage and let users become less dependent on administrators. BigQuery also helps remove the user access issues that are common with legacy data warehouses. Once that happens, users can focus on building reports, exploring datasets, and sharing trusted results easily.
3. Legacy data warehouse costs make it harder to invest in strategy.
Like other on-prem systems, data warehouses adhere to the old-school model of paying for technology, with the associated hardware and licensing costs and ongoing systems engineering. This kind of inefficient architecture drives more inefficiency. When the business is moving toward becoming data-driven, they’ll continue to ask your team for more data. But responding to those needs means you’ll run out of money pretty quickly.
Cloud offers much more cost flexibility, meaning you’re not paying for, or managing, the entire underlying infrastructure stack. Of course, it’s possible to simply port an inefficient legacy architecture into the public cloud. To avoid that, we like to talk about total cost of ownership (TCO) for data warehouses, because it captures the full picture of how legacy technology costs and business agility aren’t matching up. Moving to BigQuery isn’t just moving to cloud–it’s moving to a new cost model, where you’re cutting out that underlying infrastructure and systems engineering. You can get more detail on cloud data warehouse TCO comparisons from ESG.
4. A legacy data warehouse can’t flexibly meet business needs.
While overnight data operations used to be the norm, the global opportunities for businesses mean that a data warehouse now has to load streaming and batch data while also supporting simultaneous queries. Hardware is the main constraint for legacy systems as they struggle to keep up.
Moving your existing architecture into the cloud usually means moving your existing issues into the cloud, and we hear from businesses that doing so still doesn’t allow for real-time streaming. That’s a key component for data analysts and users. Using a platform like BigQuery means you’re essentially moving your computational capabilities into the data warehouse itself, so it scales as more and more users are accessing analytics. Unlimited compute is a pretty good way to help your business become digital. Instead of playing catch-up with user requests, you can focus on developing new features. Cloud brings added security, too, with cloud data warehouses able to do things like automatically replicate, restore and back up data, and offer ways to classify and redact sensitive data.
5. Legacy data warehouses lack built-in, mature predictive analytics solutions.
Legacy data warehouses are usually struggling to keep up with daily data needs, like providing reports to departments like finance or sales. It can be hard to imagine having the time and resources to start doing predictive analytics when provisioning and compute limits are holding your teams back.
We hear from customers that many of them are tasked with simplifying infrastructure and adding modern capabilities like AI, ML and self-service analytics for business users. The best stories about digital transformation are those where the technology changes and business or cultural changes happen at the same time. One customer told us that because BigQuery uses a familiar SQL interface, they were actually able to shift the work of data analytics away from a small, overworked group of data scientists into the hands of many more workers. Doing so also eliminated a lot of the siloed data lakes that had sprung up as data scientists extracted data one project at a time into various repositories to train ML models.
These large-scale computational possibilities save time and overhead, but also let businesses explore new avenues of growth. AI and ML are already changing the face of industries like retail, where predictive analytics can provide forecasting and other tasks to help the business make better decisions. BigQuery lets you take on sophisticated machine learning tasks without moving data or using a third-party tool.
We designed BigQuery so that our engineers deploy the resources needed for you to scale. It means your focus can change entirely toward meeting the needs the business has put forth, and bringing a lot more flexibility. BigQuery is fully serverless and runs on underlying Google infrastructure, so it integrates with our ecosystem of data and analytics partner tools. This architecture means you’re continually getting the most up-to-date software stack–analytics that scale, real-time insights, and cutting-edge functionality that includes geospatial and machine learning right from the SQL interface.
Streamline your path to data warehouse modernization with BigQuery by learning about Google Cloud’s proven migration methodology and get started with your data warehouse by applying for our migration offer.