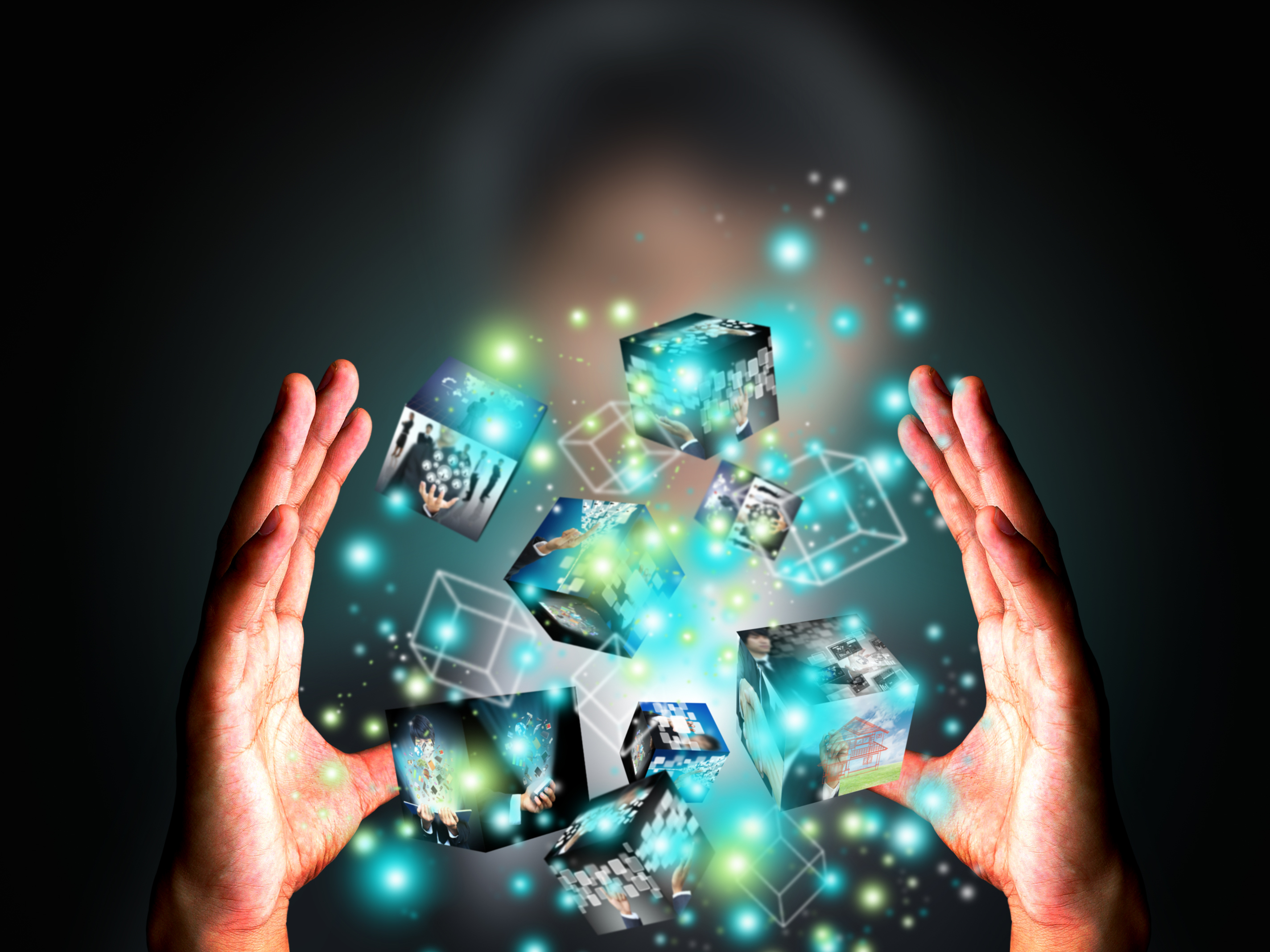
The Cybersecurity Weakest Link – Linux and IOT
April 18, 2019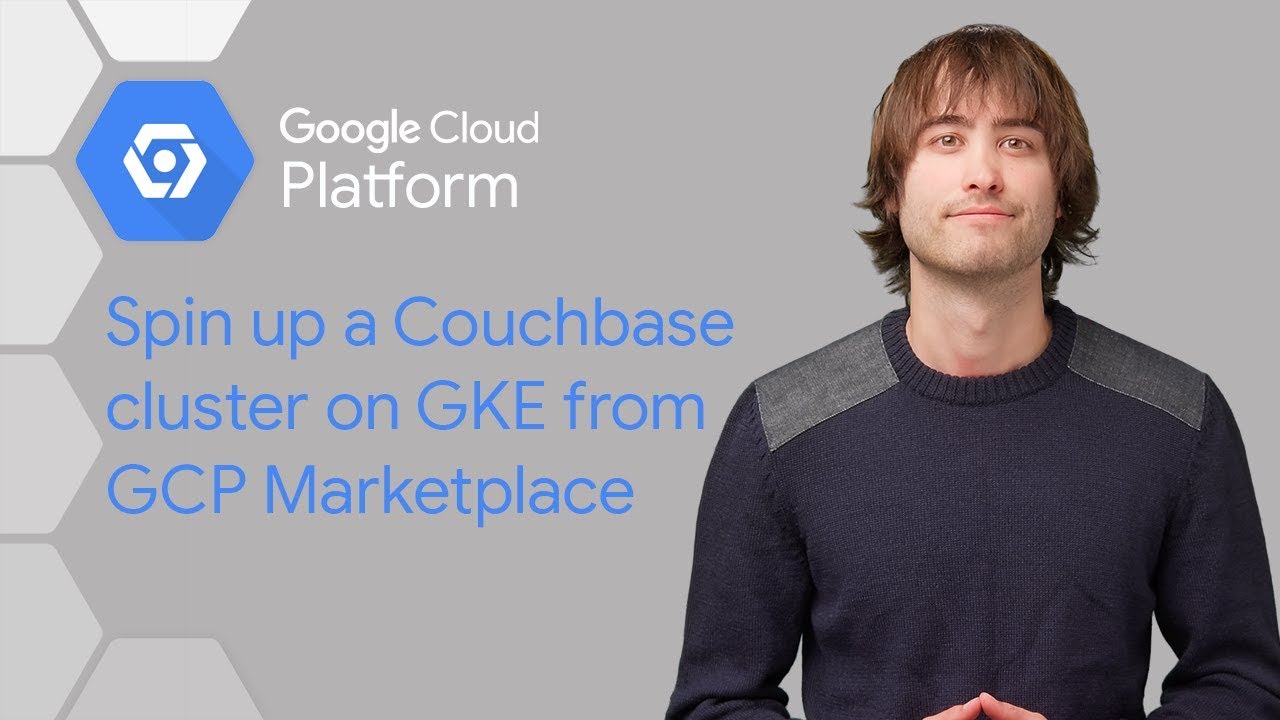
Deploy and run the Couchbase database on Kubernetes through the GCP Marketplace
April 19, 2019GKE, our cloud-hosted managed service, also supports Horizontal Pod Autoscaler and Cluster Autoscaler. But unlike open-source Kubernetes, where cluster autoscaler works with monolithic clusters, GKE uses node pools for its cluster automation. Node pools are a subset of node instances within a cluster that all have the same configuration. This lets administrators provision multiple node pools of varying machine sizes within the same cluster that the Kubernetes scheduler then uses to schedule workloads. This approach lets GKE use the right size instances from the get-go to avoid creating nodes that are too small to run some pods, or too big and waste unused compute space.
Although Horizontal Pod Autoscaler and Cluster Autoscaler are widely used on GKE, they don’t solve all the challenges that a DevOps administrator may face–pods that are over- or under-provisioned for CPU and RAM, and clusters that don’t have the appropriate nodes in a node pool with which to scale.
For those scenarios, GKE includes two advanced features: Vertical Pod Autoscaler, which automatically adjusts a pod’s CPU and memory requests, and Node Auto Provisioning, a feature of Cluster Autoscaler that automatically adds new node pools in addition to managing their size on the user’s behalf. First introduced last summer in alpha, both of these features are now in beta and ready for you to try out as part of the GKE Advanced edition, introduced earlier this week. Once these features become generally available, they’ll be available only through GKE Advanced, available later this quarter.
To better understand Vertical Pod Autoscaler and Node Auto Provisioning, let’s look at an example. Helen is a DevOps engineer in a medium-sized company. She’s responsible for deploying and managing workloads and infrastructure, and supports a team of around 100 developers who build and deploy around 50 various services for the company’s internet business.
The team deploys each of the services several times a week across dev, staging and production environments. And even though they thoroughly test every single deployment before it hits production, the services are occasionally saturated or run out of memory.
Helen and her team analyze the issues and realize that in many cases the applications go out of memory under a heavy load. This worries Helen. Why aren’t these problems caught during testing? She asks her team about how the resource requests are being estimated and assigned, but to her surprise, finds that no one really knows for sure how much CPU and RAM should be requested in the pod spec to guarantee the stability of workload. In most cases, an administrator set the memory request a long time ago and never changed it…until the application crashed, and they were forced to adjust it. Even then, adjusting the memory request isn’t always a systematic process–sometimes the admin regularly tests the app under heavy load, but more often they simply add some more memory. How much memory exactly? Nobody knows.
In some ways, the Kubernetes CPU and RAM allocation model is a bit of a trap: Request too much and the underlying cluster is less efficient; request too little and you put the entire service at risk. Helen checks the GKE documentation and discovers Vertical Pod Autoscaler.
Vertical Pod Autoscaler is inspired by a Google Borg service called AutoPilot. It does three things:
1. It observes the service’s resource utilization for the deployment.
2. It recommends resource requests.
3. It automatically updates the pods’ resource requests, both for new pods as well as for current running pods.