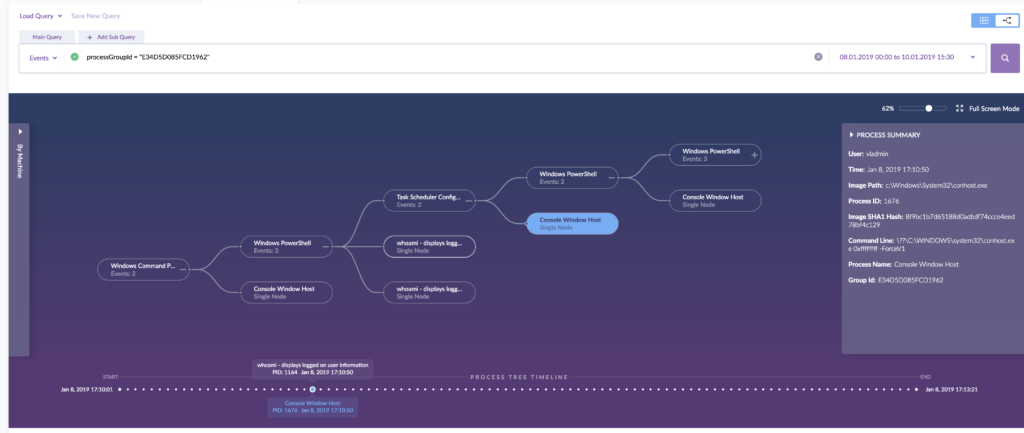
RSAC DAY 2 – Intel Inside, Threat Hunting and Dancing around with Friends
March 6, 2019
The service mesh era: Using Istio and Stackdriver to build an SRE service
March 6, 2019When you’re copying or moving data between distinct storage systems such as multiple Apache Hadoop Distributed File System (HDFS) clusters or between HDFS and Cloud Storage, it’s a good idea to perform some type of validation to guarantee data integrity. This validation is essential to be sure data wasn’t altered during transfer.
For Cloud Storage, this validation happens automatically client-side with commands like gsutil cp
and rsync
. Those commands compute local file checksums, which are then validated against the checksums computed by Cloud Storage at the end of each operation. If the checksums do not match, gsutil deletes the invalid copies and prints a warning message. This mismatch rarely happens, and if it does, you can retry the operation.
Now, there’s also a way to automatically perform end-to-end, client-side validation in Apache Hadoop across heterogeneous Hadoop-compatible file systems like HDFS and Cloud Storage. Our Google engineers recently added the feature to Apache Hadoop, in collaboration with Twitter and members of the Apache Hadoop open-source community.
While various mechanisms already ensure point-to-point data integrity in transit (such as TLS for all communication with Cloud Storage), explicit end-to-end data integrity validation adds protection for cases that may go undetected by typical in-transit mechanisms. This can help you detect potential data corruption caused, for example, by noisy network links, memory errors on server computers and routers along the path, or software bugs (such as in a library that customers use).
In this post, we’ll describe how this new feature lets you efficiently and accurately compare file checksums.
How HDFS performs file checksums
HDFS uses CRC32C, a 32-bit Cyclic Redundancy Check (CRC) based on the Castagnoli polynomial, to maintain data integrity in several different contexts:
- At rest, Hadoop DataNodes continuously verify data against stored CRCs to detect and repair bit-rot.
- In transit, the DataNodes send known CRCs along with the corresponding bulk data, and HDFS client libraries cooperatively compute per-chunk CRCs to compare against the CRCs received from the DataNodes.
- For HDFS administrative purposes, block-level checksums are used for low-level manual integrity checks of individual block files on DataNodes.
- For arbitrary application-layer use cases, the
FileSystem
interface definesgetFileChecksum
, and the HDFS implementation uses its stored fine-grained CRCs to define such a file-level checksum.
For most day-to-day uses, the CRCs are used transparently with respect to the application layer, and the only CRCs used are the per-chunk CRC32Cs, which are already precomputed and stored in metadata files alongside block data. The chunk size is defined by dfs.bytes-per-checksum
and has a default value of 512 bytes.
Shortcomings of Hadoop’s default file checksum type
By default when using Hadoop, all API-exposed checksums take the form of an MD5 (a message-digest algorithm that produces hash values) of a concatenation of chunk CRC32Cs, either at the block level through the low-level DataTransferProtocol
, or at the file level through the top-level FileSystem
interface. The latter is defined as the MD5 of the concatenation of all the block checksums, each of which is an MD5 of a concatenation of chunk CRCs, and is therefore referred to as an MD5MD5CRC32FileChecksum
. This is effectively an on-demand, three-layer Merkle tree.
This definition of the file-level checksum is sensitive to the implementation and data-layout details of HDFS, namely the chunk size (default 512 bytes) and the block size (default 128MB). So this default file checksum isn’t suitable in any of the following situations:
- Two different copies of the same files in HDFS, but with different per-file block sizes configured.
- Two different instances of HDFS with different block or chunk sizes configured.
- Copying across non-HDFS Hadoop-compatible file systems
- https://wiki.apache.org/hadoop/HCFS
- (HCFS) such as Cloud Storage.
You can see here how the same file can end up with three checksums depending on the file system’s configuration: